Predictive Analytics and AI: Forecasting Ad Success With Accuracy
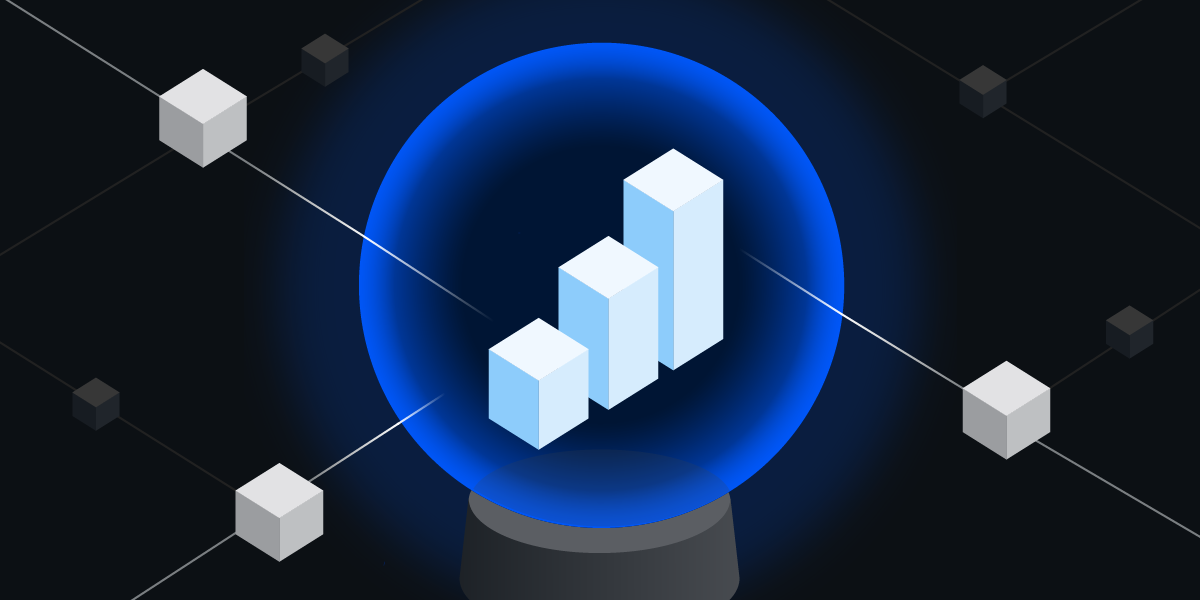
Brands need more than guesswork to reach the right audience at the right time. That’s where predictive analytics comes in.
Predictive analytics anticipates consumer behaviour, helping advertisers make smarter decisions and maximize their return on investment.
AI has become a game-changer in the field, enhancing campaign performance by analyzing vast amounts of real-time data. It allows advertisers to optimize ad placements, refine audience targeting, and adjust bidding strategies with unprecedented precision.
With AI-powered predictive models, brands can target their ads to reach the most relevant consumers at the most effective moments.
Forecasting is critical to ad success because it allows advertisers to anticipate trends, budget efficiently, and improve conversion rates. Instead of relying on past performance alone, predictive analytics empowers marketers with forward-looking insights, helping them adapt to changing consumer behaviours and market dynamics.
As AI continues to evolve, predictive analytics will play an even bigger role in shaping the future of digital advertising.
The Role of Machine Learning in Predictive Advertising
Machine learning has transformed digital advertising by enabling real-time decision-making that maximizes return on investment. It analyzes vast amounts of data to predict which ads are most likely to drive engagement, clicks, and conversions, helping advertisers allocate their budgets more effectively.
Machine learning is crucial in determining ad success by evaluating every opportunity to bid in an auction.
Ad auction predictions and bid-time decisions are at the core of AI-driven advertising platforms. Each time an ad impression becomes available, platforms such as StackAdapt must decide within milliseconds whether to bid on it and how much to pay.
Machine learning models assess multiple factors, including the likelihood of a user clicking or converting, before making an informed bid decision. Optimizing bids in real time ensures that budgets are spent on high-value opportunities rather than wasted on low-impact impressions.
One of the biggest challenges in predictive advertising is balancing speed and accuracy. Machine learning models must make precise calculations almost instantaneously, as ad auctions operate on millisecond-level timeframes.
Unlike large AI models that take several seconds to generate an output, predictive analytics in advertising requires highly optimized algorithms capable of delivering both speed and accuracy.
Predictions that take too long result in lost bidding opportunities, while inaccurate predictions can lead to overpaying for ineffective impressions.
Continuous refinement of these models allows AI-driven platforms to improve campaign performance and ensure that every advertising dollar is used efficiently.
Key Data Sources for Predictive Analytics
Effective predictive analytics in advertising relies on high-quality data. The accuracy of AI-driven forecasts depends on the sources and reliability of the data used to train models and inform decisions.
Three primary data sources play a crucial role in predictive advertising: 1st-party data, 3rd-party data, and historical campaign data.
First-party data serves as the foundation of predictive modelling. Every brand has unique customer interactions, purchase histories, and behavioural patterns that can be leveraged to optimize advertising strategies.
This data, collected directly from customer interactions on websites, apps, and CRM systems, offers the most accurate and relevant insights. Because it reflects actual consumer behaviour, 1st-party data allows advertisers to create highly personalized campaigns and improve targeting precision.
Third-party data provides additional layers of insight but comes with quality, accuracy, and integration challenges. Advertisers often rely on 3rd-party providers to supplement their data, gaining access to broader audience segments, demographic details, and interest-based targeting.
However, not all 3rd-party data is equally reliable, and its effectiveness depends on the source’s credibility, the data’s freshness, and how well it aligns with 1st-party insights. Due diligence is necessary to ensure that 3rd-party data enhances, rather than distorts, predictive models.
Historical campaign data plays a key role in refining ad strategies. Past performance trends reveal patterns in user engagement, conversion rates, and ad effectiveness, helping advertisers fine-tune future campaigns.
Analyzing factors such as seasonality, audience behaviour over time, and the effectiveness of different creative approaches allows predictive models to improve over successive campaigns.
This iterative learning process ensures that advertising efforts become more efficient, maximizing return on investment while reducing wasted ad spend.
AI-Driven Consumer Behaviour Predictions
Predicting consumer behaviour has always been challenging in advertising, but AI has significantly improved the ability to anticipate decision-making patterns and optimize campaigns accordingly. AI can identify when and how consumers will likely convert, allowing advertisers to refine targeting strategies and improve ad performance.
AI helps track decision-making timelines and conversion patterns. Instead of relying on general assumptions about the customer journey, predictive analytics can assess how many ads a user sees, over what period, and how long it typically takes them to make a purchase decision.
This insight allows advertisers to adjust their budgets so campaigns remain effective throughout the buying cycle.
AI can also detect shifts in consumer timelines, such as longer decision-making periods due to economic factors or seasonal trends, enabling brands to respond proactively.
Personalized advertising has also become more precise with AI. Instead of serving generic ads to broad audiences, AI enables real-time personalization by analyzing user preferences, past interactions, and browsing behaviour.
Ads can be tailored based on location, product interest, and engagement history, increasing the likelihood of conversion.
Understanding incremental lift and cross-channel attribution is another critical aspect of AI-driven consumer behaviour predictions.
- Incremental lift measures the actual impact of advertising by determining whether an ad influenced a consumer’s decision or if they would have converted regardless. AI can analyze performance across multiple channels, from display and search to social media and video, identifying which touchpoints contribute most to conversions.
- Cross-channel attribution ensures that advertising budgets are allocated effectively by recognizing each channel’s role in guiding consumers toward a purchase.
Overcoming Challenges in Predictive Advertising
Predictive advertising offers powerful insights, but several challenges must be addressed to ensure effectiveness. Data accuracy, real-time processing, and privacy regulations are critical factors influencing how well machine learning models can predict consumer behaviour and optimize ad performance.
Challenge | Description | Next Steps |
Ensuring Data Accuracy and Verification | Predictive models rely on high-quality input data to generate meaningful outputs. Verification processes help ensure data consistency between training and real-world conditions, reducing the risk of misleading predictions. | Invest in data verification. |
Processing Massive Data Volumes in Real Time | Ad auctions operate on millisecond timeframes, requiring machine learning models to analyze vast datasets and make split-second decisions. Optimization is crucial to prevent excessive costs and missed opportunities. | Optimize real-time processing. |
Navigating Privacy Regulations and Ethical Considerations | Evolving privacy laws, such as GDPR and CCPA, restrict data collection methods. Companies must adopt alternative strategies while maintaining transparency and respecting user privacy. | Stay ahead of regulatory changes to maximize the benefits of predictive analytics while maintaining consumer trust. |
Real-World Applications of Predictive Analytics in Advertising
From weather-driven ad targeting to automated budget allocation, AI is being used innovatively to improve ad performance and maximize return on investment.
AI-powered weather-based ad targeting has become a crucial tool for industries that rely on seasonal demand.
For example, in home services businesses, such as HVAC, roofing, and plumbing companies, predictive analytics can adjust advertising strategies based on weather patterns. AI-driven models analyze forecast data and trigger specific ad campaigns when certain conditions are met. If a cold front is expected, ads for heating services can be prioritized in affected regions.
Similarly, ads for flood prevention or emergency repairs can be dynamically adjusted in areas anticipating storms.
Automated ad spend allocation across multiple channels is another major advancement in AI-driven advertising. Predictive models continuously assess campaign performance across different platforms, such as display, search, and social media.
Instead of relying on manual adjustments, AI dynamically shifts budgets toward the most effective channels in real time. If data shows that social media ads drive higher engagement for a particular audience segment, more funds can be allocated while reducing spending on underperforming channels.
Case studies of brands successfully leveraging predictive analytics further demonstrate the impact of AI in advertising. Companies using in-house predictive models or AI-powered platforms like StackAdapt have been able to fine-tune their targeting and attribution strategies.
Some brands run detailed A/B tests and incrementality studies to measure the true impact of their campaigns, ensuring that ad spend results in meaningful growth rather than wasted impressions.
Others have integrated predictive analytics into their media mix modelling, identifying the best-performing ad formats and adjusting creatives in response to user engagement patterns.
The Future of Predictive Analytics and AI in Advertising
As brands refine their data strategies, AI-driven marketing platforms evolve to provide more integrated, automated, and intelligent solutions. The future of predictive analytics lies in seamless AI integration, enhanced personalization, and greater accessibility for businesses of all sizes.
The industry is shifting towards integrated AI-driven marketing platforms. Many companies rely on fragmented tools and multiple data sources to manage advertising efforts. AI is helping to bridge these gaps by creating end-to-end platforms that unify 1st-party data, 3rd-party insights, media buying, and performance analytics.
Combining these elements provides a more comprehensive view of customer behaviour, leading to more accurate forecasting and improved ad spend allocation.
Marketers no longer need to manually connect insights across different platforms, as AI-driven ecosystems are automating much of this process to make predictive analytics more efficient and actionable.
Personalization and performance measurement continue to evolve as AI technology advances. Advertising will move beyond basic audience segmentation, with AI models dynamically adjusting ad creatives, messaging, and delivery in real time based on individual user behaviours and preferences.
Performance measurement is also becoming more precise, shifting away from traditional last-click attribution models toward incrementality-based analytics that assess the true impact of advertising efforts.
A deeper understanding of causation in consumer actions ensures that marketing decisions are based on real influence rather than surface-level correlations.
Small and medium-sized businesses (SMBs) are gaining the ability to leverage AI without requiring massive data sets. AI-driven advertising has traditionally favoured large brands with extensive data resources, making it difficult for smaller businesses to compete.
But modern AI platforms can now operate effectively with smaller datasets, using pre-trained models and lookalike audience strategies to deliver accurate predictions.
Advertising platforms like StackAdapt offer built-in AI tools that SMBs can use immediately, reducing the need for expensive, custom-built data infrastructure.
Even businesses with relatively low customer interaction volumes can benefit from AI-driven insights, optimizing ad spend, improving targeting, and enhancing campaign performance without requiring a dedicated data science team.
Predictive analytics and AI in advertising will continue advancing toward greater automation, deeper insights, and increased accessibility.
As AI-powered tools evolve, businesses of all sizes will have the opportunity to adopt data-driven advertising strategies that improve efficiency, maximize return on investment, and remain competitive in an increasingly AI-driven marketing landscape.
Are you ready to use AI and predictive analytics in your next campaign? Talk to us about how StackAdapt can help you reach your goals.